Bilytica # 1 is one of the top Business Intelligence Analyst as it forms the foundation for reliable insights and informed decision-making within an organization. In this article, we delve into the strategies and best practices that BI analysts employ to maintain data accuracy throughout the data lifecycle.
Click to Start Whatsapp Chat with Sales
Call #:+923333331225
Email: sales@bilytica.com
Bilytica #1 Business Intelligence Analyst
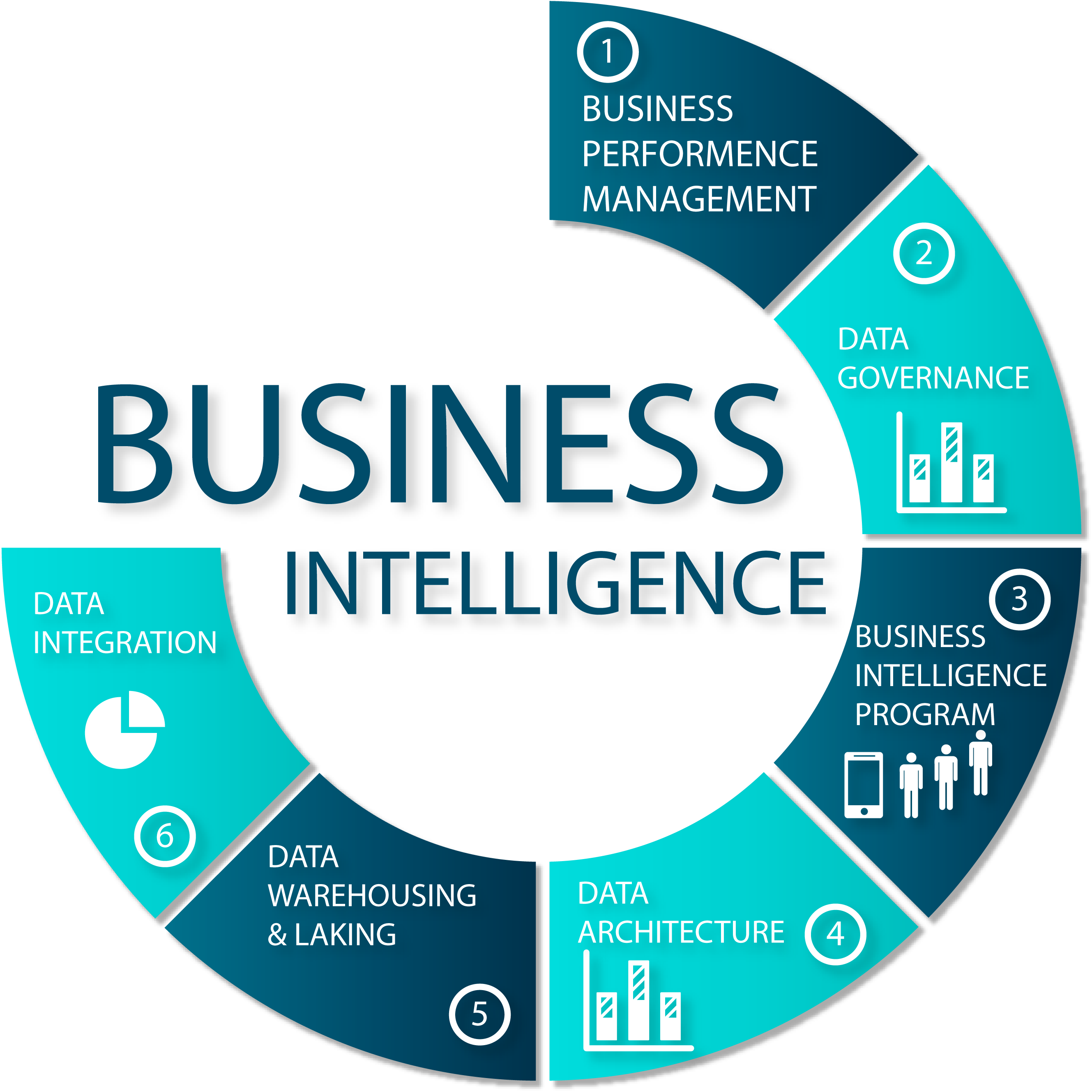
Understanding Data Sources
The first step in ensuring Business Intelligence Analyst is to thoroughly understand the data sources being utilized. This involves:
- Source Evaluation: Evaluating the reliability and credibility of data sources to determine their suitability for analysis. BI analysts assess factors such as data quality, data freshness, and the reputation of the source.
- Data Profiling: Conducting data profiling activities to gain insights into the structure, content, and quality of the data. This includes identifying anomalies, inconsistencies, and missing values within the dataset.
Implementing Data Validation Checks
Data validation checks are essential for identifying errors, inconsistencies, and discrepancies within the dataset. BI analysts employ various techniques to validate the accuracy of the data:
- Completeness Checks: Verifying that all required data fields are populated and that no values are missing or null. This ensures that the dataset is complete and suitable for analysis.
- Consistency Checks: Ensuring that data values are consistent across different data sources and data elements. BI analysts compare data values against predefined rules or reference datasets to identify inconsistencies.
- Accuracy Checks: Validating the accuracy of data values by comparing them against known benchmarks, historical data, or external sources. This helps identify errors or outliers that may skew the analysis.
- Integrity Checks: Verifying the integrity of relationships between data elements to ensure data coherence and reliability. BI analysts validate referential integrity constraints and foreign key relationships to maintain data integrity.
Standardizing Data Formats
Standardizing data formats and conventions is essential for maintaining consistency and accuracy across the dataset:
- Data Standardization: Converting data values into a standardized format to ensure uniformity and comparability. This may involve standardizing date formats, unit conversions, and categorical values.
- Normalization: Normalizing data values to eliminate redundancy and duplication, thereby reducing the risk of errors and inconsistencies. BI analysts employ techniques such as database normalization to organize data into logical structures.
- Data Governance Policies: Establishing data governance policies and standards to enforce consistency and accuracy in data management practices. BI analysts define data naming conventions, data dictionaries, and data validation rules to ensure adherence to standards.
Data Cleaning and Enrichment
Data cleaning and enrichment techniques are employed to enhance the accuracy and completeness of the dataset:
- Data Cleaning: Removing errors, inconsistencies, and outliers from the dataset through data cleansing techniques. Business Intelligence Analyst use tools such as data deduplication, outlier detection, and error correction algorithms to clean the data.
- Data Imputation: Filling in missing values or correcting erroneous values through data imputation techniques. BI analysts employ statistical methods such as mean imputation, regression imputation, and nearest neighbor imputation to impute missing data values.
- Data Enrichment: Enhancing the dataset with additional attributes or information to enrich the analysis. BI analysts integrate external data sources, such as demographic data, market research reports, and geospatial data, to provide context and depth to the analysis.
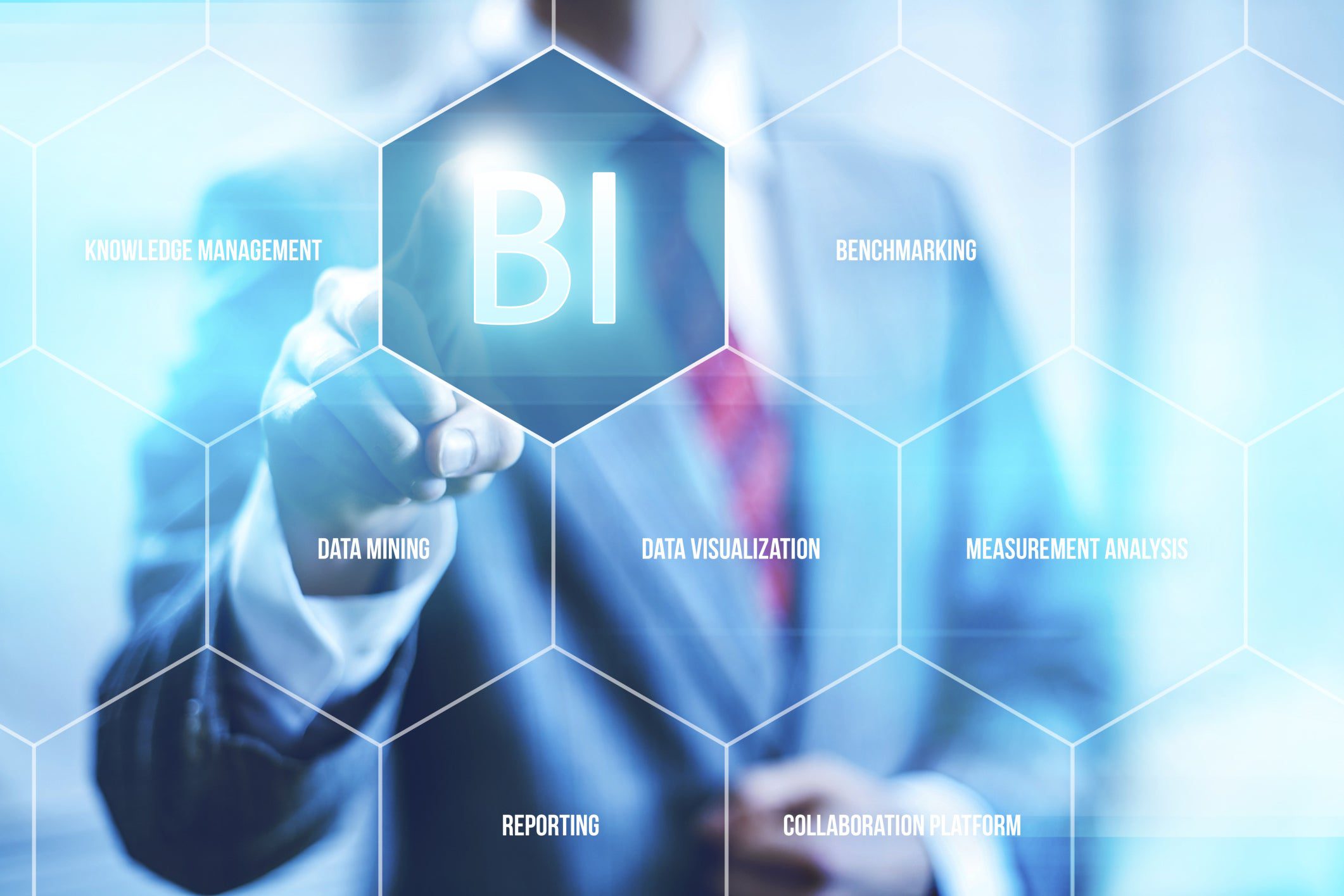
Implementing Robust Data Governance Practices
Data governance practices play a critical role in ensuring data accuracy and reliability:
- Data Quality Management: Establishing data quality management processes to monitor, measure, and improve data quality. Business Intelligence Platform define data quality metrics, conduct data quality assessments, and implement corrective actions to address data quality issues.
- Data Stewardship: Assigning data stewardship responsibilities to individuals or teams within the organization to oversee data quality and integrity. Data stewards are responsible for data profiling, data cleansing, and data validation activities.
- Data Security: Implementing robust data security measures to protect data integrity and confidentiality. BI analysts ensure that data access controls, encryption protocols, and data masking techniques are in place to safeguard sensitive information.
Continuous Monitoring and Maintenance
Maintaining data accuracy is an ongoing process that requires continuous monitoring and maintenance:
- Data Auditing: Conducting regular audits and reviews of the dataset to identify data anomalies, inconsistencies, and errors. BI analysts perform data audits to ensure that data accuracy standards are met and maintained.
- Automated Alerts: Implementing automated alerting mechanisms to notify stakeholders of potential data quality issues or anomalies in real-time. BI analysts set up automated alerts for data quality thresholds, data validation failures, and data integrity breaches.
- Data Refreshes: Ensuring that data sources are regularly updated and refreshed to reflect changes and updates. Power BI schedule data refreshes to ensure that the dataset remains current and accurate, especially for time-sensitive analyses.
Conclusion
Ensuring data accuracy is essential for the success of BI initiatives and data-driven decision-making within organizations. By employing a combination of strategies such as understanding data sources, implementing validation checks, standardizing data formats, cleaning and enriching data, implementing robust data governance practices, and continuous monitoring and maintenance, BI analysts can maintain high levels of data accuracy throughout the data lifecycle. This not only enhances the reliability of insights derived from the data but also instills confidence in decision-makers relying on BI outputs.
Click to Start Whatsapp Chat with Sales
Call #:+923333331225
Email: sales@bilytica.com
Business Intelligence Analyst
Business Intelligence Analyst
Business Intelligence Analyst
20-5-2024